Kandinsky v2.1
Exploring Kandinsky V2.1 Text to Image Diffusion model
Kandinsky v2.1 is a text2image latent diffusion model, inheriting best practices from its predecessors, DALL-E 2 and Latent Diffusion. Additionally, it introduces new ideas for text-guided image manipulation and image fusion (interpolation).
Kandinsky 2.1 uses CLIP for encoding and a diffusion image prior for mapping between CLIP modalities. This improves image quality and manipulation capabilities, unlike other multilingual models with their own version of CLIP.
Kandinsky 2.1 Architecture key Details
- Transformer (num_layers=20, num_heads = 32 and hidden_size = 2048)
- Text Encoder (XLM-Roberta-Large-Vit-L-14)
- Diffusion Image Prior
- CLIP Image Prior
- CLIP Image Encoder (VIT-L/14)
- Latent Diffusion U-Net
- MoVQ encoder / decoder
Kandinsky 2.1 works extremely well with creative prompts, as it understands the input prompts a lot better than Stable Diffusion. This is mainly because it uses the same CLIP Encoder as DALLE-2 when it comes to text encoding.
It encodes text prompts with CLIP. Then, it diffuses the CLIP text embeddings to CLIP image embeddings. Finally, it uses the image embeddings for image generation.

Conclusion and Competing with State of the Art
Following the successful deployment of Kandinsky 2.1, the AI Forever team has achieved notable advancements across various domains of applied image synthesis. These achievements encompass image blending, textual description-driven image manipulation, and both inpainting and outpainting techniques. Building upon these accomplishments, the team at Kandinsky aims to enhance the capabilities of its image encoder, fortify the text encoder, and explore the realm of higher-resolution image generation. The iterative refinement of Kandinsky signifies a relentless pursuit of pushing the frontiers of image synthesis. Recently, the team has introduced Kandinsky V2.2 and Kandinsky V3 models, which represent iterative improvements upon the existing architecture, marking further progress in this evolving field.
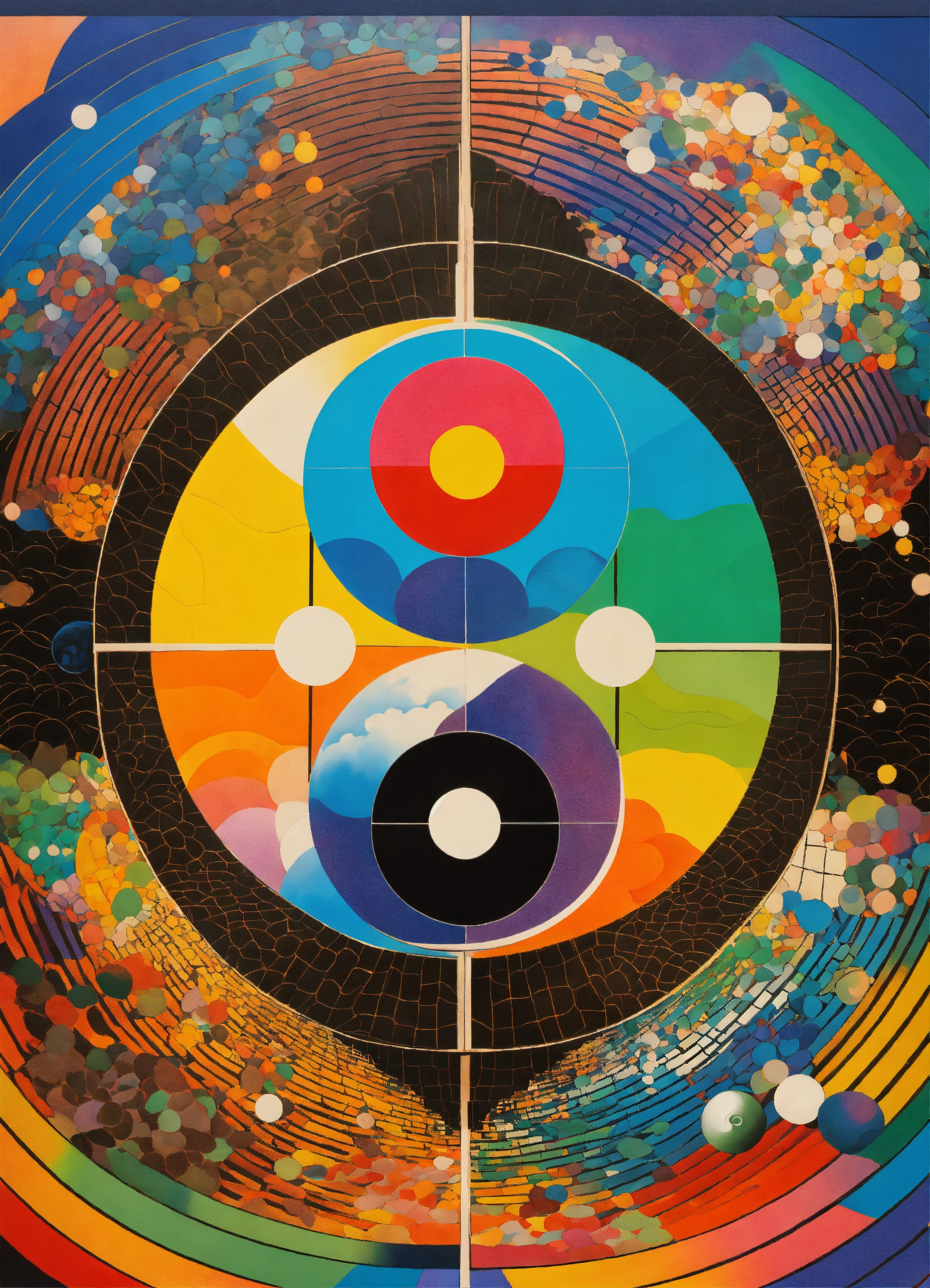